- Published on
Tesla's Optimus Learns New Tricks: Autonomously Performing Chores by Watching Humans
- Authors
- Name
- The Editorial Team
- @humanoidsdaily
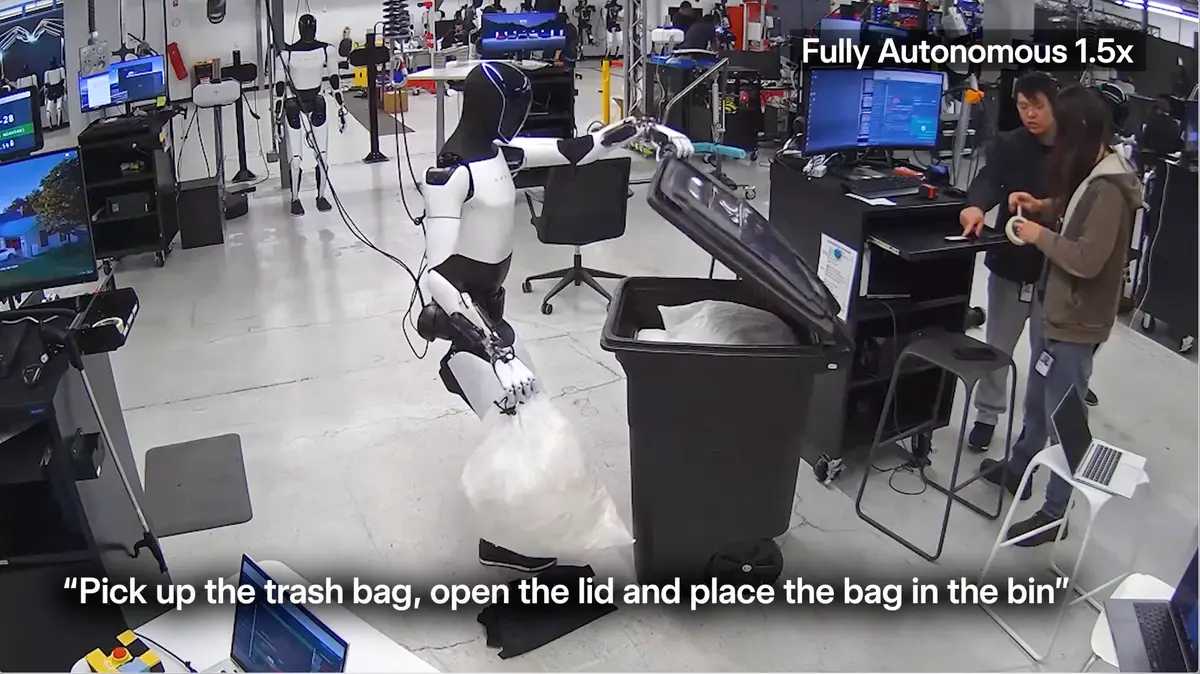
Optimus Adopts Human-See, Human-Do Approach for Faster Task Learning
Tesla has released a new video demonstrating its Optimus humanoid robot performing a variety of tasks autonomously, reportedly learned by observing humans. The company claims this breakthrough significantly speeds up the process of teaching the robot new skills, relying on a single neural network to interpret natural language instructions and execute actions like tidying up, operating appliances, and handling objects.
I’m not just dancing all day, ok
The video, accompanied by statements from CEO Elon Musk and members of the Optimus team, shows the robot picking up a trash bag and placing it in a bin, cleaning a table with a brush and dustpan, tearing a paper towel, stirring a pot, vacuuming, and even handling specific automotive parts. Text overlays in the video state, "All these tasks are done by a single neural net and were learned directly from human videos. This breakthrough allows us to learn new tasks much faster & we're now working on further improving reliability."
A Leap in Learning Methodology
Milan Kovac, VP and Head of Engineering for Optimus, elaborated on the development, stating, "One of our goals is to have Optimus learn straight from internet videos of humans doing tasks." He explained that the team recently achieved a "significant breakthrough" enabling them to "transfer a big chunk of the learning directly from human videos to the bots (1st person views for now)." This approach is intended to "bootstrap new tasks much faster compared to teleoperated bot data alone," which Kovac described as "heavier operationally."
One of our goals is to have Optimus learn straight from internet videos of humans doing tasks. Those are often 3rd person views captured by random cameras etc. We recently had a significant breakthrough along that journey, and can now transfer a big chunk of the learning
I’m not just dancing all day, ok
Ashish Kumar, leading AI for Tesla Optimus, emphasized that "All tasks are from the same neural net that understands text instructions!" and reiterated that the "technical breakthrough is in directly learning from first person videos of humans doing tasks!" Mihir Dalal, also on the Optimus AI team, highlighted the significance, noting, "We can now do bi-manual, dexterous manipulation across a wide range of tasks with barely any data on these skills coming from teleoperation. As we know, teleop does not scale! But turns out human video does!"
Tesla's next steps include expanding this learning capability to encompass third-person view videos, akin to random internet footage, and enhancing reliability through reinforcement learning (RL) in both real-world and simulated environments.
Broader Trends in Robotic Learning
This development at Tesla occurs amidst a broader surge in advancements within reinforcement learning for humanoid robotics. Recently, NVIDIA detailed its Isaac GR00T N1.5 foundation model and the "GR00T-Dreams" system, which generates synthetic motion data by leveraging video diffusion models. This system can take a single image input and produce videos of robots performing new tasks, which are then processed into "action tokens" for training. NVIDIA's approach, as outlined by its Director of AI, Jim Fan, focuses on overcoming data bottlenecks through simulation, progressing from "Digital Twins" to "Digital Nomads" that can learn from AI-imagined scenarios.
While Tesla is now emphasizing learning from direct human video, and NVIDIA is exploring AI-generated video for synthetic data, both strategies underscore the critical role of sophisticated data pipelines and RL in pushing humanoid capabilities forward. The goal for many in the field is to create robots that can adapt to new situations and learn continuously.
Despite the impressive demonstrations, the path to truly general-purpose humanoid robots that can reliably operate in unstructured human environments remains challenging. Ensuring that skills learned, whether from human videos or synthetic data, translate robustly to diverse real-world scenarios is a persistent hurdle. Tesla acknowledges the ongoing work to improve reliability, with team members pointing to RL as the next frontier for this.
The rapid pace of development, showcased by Tesla, NVIDIA, and others in the academic and commercial sectors, indicates a vibrant and highly competitive landscape. As these humanoid platforms mature, the focus will increasingly shift from demonstrating individual skills to achieving consistent, reliable performance in practical applications.