- Published on
NVIDIA Advances Humanoid AI with GR00T N1.5, Eyeing a Future of Simulated Realities
- Authors
- Name
- Humanoids daily
- @humanoidsdaily
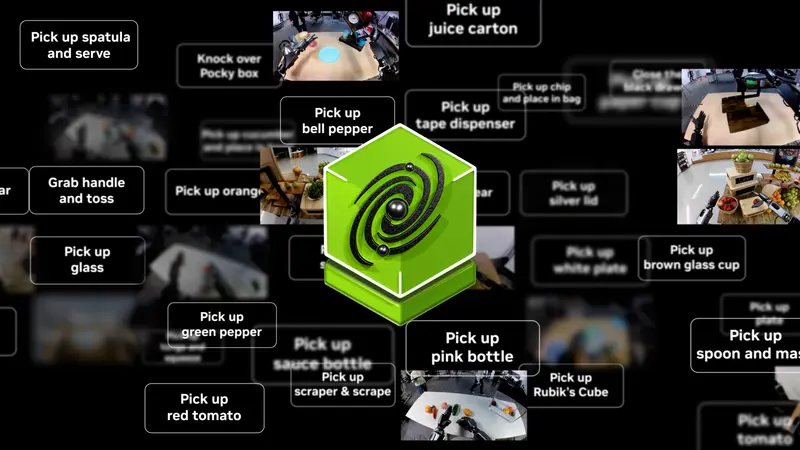
NVIDIA has announced a significant update to its humanoid robot foundation model, Isaac GR00T N1.5, alongside a suite of new tools aimed at accelerating the development of "Physical AI." The announcements, made at COMPUTEX, highlight the company's strategy to overcome the immense data challenges in robotics through advanced simulation and synthetic data generation, a vision recently detailed by NVIDIA's Director of AI, Jim Fan.
GR00T Grows: NVIDIA Unveils Enhanced Humanoid Model and Data Generation Tools
NVIDIA is pushing forward in the race to create more capable and adaptable humanoid robots with the release of Isaac GR00T N1.5. This first update to its open, generalized foundation model aims to improve humanoid reasoning and skills, particularly in adapting to new environments and understanding user instructions for tasks like material handling.
A key enabler for this rapid iteration is the new "GR00T-Dreams" blueprint. This system focuses on generating vast amounts of synthetic motion data, or "neural trajectories." According to NVIDIA, this allows developers to teach robots new behaviors and adapt to changing environments more efficiently. The company claims that GR00T N1.5 was developed in just 36 hours using synthetic training data from GR00T-Dreams, a task that would have purportedly taken nearly three months with manual data collection.
This addresses a core problem in robotics: the data bottleneck. As Jim Fan, NVIDIA's Director of AI & Distinguished Scientist, articulated in a recent AI Ascent 2025 talk, real-world robot data collection is akin to "burning human fuel"—slow, expensive, and limited. "You cannot scrape [robot joint control signals] from the internet," Fan stated, emphasizing the stark contrast with the data-rich environments available for training Large Language Models.
GR00T-Dreams complements the existing GR00T-Mimic blueprint, which augments existing data. While Mimic uses NVIDIA Omniverse and Cosmos platforms for data augmentation, Dreams leverages Cosmos to generate entirely new datasets from a single image input, producing videos of robots performing new tasks in novel settings. These videos are then processed to extract "action tokens" for robot training.
The Simulation Ladder: From Digital Twins to Digital Nomads
Fan's talk provided a conceptual framework for understanding NVIDIA's multi-pronged approach to simulation. He described a progression:
- Simulation 1.0: Digital Twins. This involves creating a one-to-one, high-fidelity copy of the robot and its environment using classical, vectorized physics engines. These simulations can run thousands of times faster than real-time, enabling rapid training for tasks like walking or dexterous manipulation through "domain randomization"—varying parameters like gravity and friction. Fan highlighted that a mere 1.5 million parameters were sufficient for a neural network to achieve complex whole-body control in humanoids, transferred zero-shot to real robots.
- The Intermediate Step: Digital Cousins. As developers move beyond precise digital twins, they enter the realm of "Digital Cousins." Here, environments are procedurally generated using 3D assets from generative models and textures from diffusion models, composed via LLMs. This allows for greater diversity in training scenarios, as showcased by NVIDIA's Robocasa framework for everyday tasks. Human teleoperation in these simulated environments can then be multiplied by generating variations in motion and environment, significantly amplifying the utility of a single demonstration.
- Simulation 2.0: Digital Nomads. The most advanced stage involves "Digital Nomads" wandering into the "dream space" of video diffusion models. By fine-tuning state-of-the-art video generation models on real robot lab data, NVIDIA can prompt these models to imagine and simulate complex, counterfactual scenarios—robots interacting with diverse objects, fluids, and soft bodies—that would be incredibly challenging for classical physics engines. Fan showed examples where a model, given the same initial frame, could generate different video outcomes based on language prompts. "The video diffusion model doesn't care how complex the scene is," Fan noted, calling it a "simulation of the multiverse."
This "embodied scaling law," as Fan termed it, suggests that combining the speed of classical simulation with the diversity of neural world models offers a "nuclear power" to scale robotics development, though it will demand ever-increasing compute resources.
Expanding the Toolkit for Physical AI
Alongside GR00T N1.5 and Dreams, NVIDIA announced several other technologies to bolster the robotics development pipeline:
- NVIDIA Cosmos Reason: A new World Foundation Model (WFM) using chain-of-thought reasoning to curate higher-quality synthetic data, now available on Hugging Face.
- Cosmos Predict 2: An upcoming WFM with performance enhancements for world generation and reduced hallucination.
- NVIDIA Isaac Sim™ 5.0: The simulation and synthetic data generation framework will soon be openly available on GitHub.
- NVIDIA Isaac Lab 2.2: An open-source robot learning framework, updated to support new evaluation environments for GR00T N models.
- Open-Source Physical AI Dataset: Now includes 24,000 high-quality humanoid robot motion trajectories used for GR00T N model development.
This comprehensive suite aims to lower the barrier to entry and accelerate innovation in the field. Companies like Agility Robotics, Boston Dynamics, Foxconn, Fourier, Lightwheel, NEURA Robotics, and XPENG Robotics are already adopting various NVIDIA Isaac platform technologies.
The "Physical Turing Test" and the Road Ahead
The ultimate goal, as Fan envisions it, is to pass the "Physical Turing Test"—a scenario where one cannot distinguish if a complex series of physical tasks (like cleaning a messy room and preparing a meal) was performed by a human or a machine. Achieving this requires robots that can perceive, reason, and act with a high degree of autonomy and adaptability.
NVIDIA's strategy, heavily reliant on simulation and powerful AI models, is a significant step in this direction. The company is also providing the hardware backbone, with global manufacturers building NVIDIA RTX PRO 6000 workstations and servers based on the Blackwell architecture, and the upcoming Jetson Thor platform for on-robot inference.
However, the path to truly general-purpose humanoid robots remains challenging. While simulation offers a powerful way to generate data, the sim-to-real gap—ensuring that behaviors learned in simulation transfer effectively to the physical world—continues to be an active area of research. The complexity of real-world physics, sensory noise, and unexpected interactions present ongoing hurdles.
NVIDIA's latest announcements underscore a strong belief that the combination of sophisticated foundation models, scalable simulation, and powerful compute is the key to unlocking the next industrial revolution powered by Physical AI. The industry will be watching closely to see how these tools translate into real-world robotic capabilities and whether the "Physical Turing Test" moves from a far-off concept to an achievable milestone, perhaps, as Fan mused, on "just another Tuesday."
Watch Dr Jim Fan's talk here: